Artificial intelligence (AI) and related technologies are increasingly prevalent in business and society, and are beginning to be applied to healthcare. These technologies have the potential to transform many aspects of patient care, as well as administrative processes within provider, payer, and pharmaceutical organizations. AI in healthcare management is at the forefront of innovation, offering solutions that can improve performance and reduce costs.
Key Takeaways:
- AI has the potential to revolutionize healthcare by improving care outcomes and enhancing the productivity and efficiency of care delivery.
- AI can automate aspects of care, freeing up healthcare professionals’ time to focus on patient care.
- The key categories of AI applications in healthcare include diagnosis and treatment recommendations, patient engagement and adherence, and administrative activities.
- Current research studies show that AI can perform healthcare tasks as well or better than humans in certain areas.
- Ethical considerations and challenges need to be addressed to ensure responsible and ethical application of AI in healthcare.
- Machine learning, natural language processing, rule-based expert systems, and physical robots are some of the AI technologies being used in healthcare.
- AI can help healthcare systems overcome financial pressures and improve their overall performance.
- Challenges to AI adoption in healthcare include implementation factors, lack of awareness, and resistance to change.
- The impact of AI on healthcare professionals and their roles in the industry is a topic of discussion and exploration.
- The future of AI in healthcare holds great promise for transformative advancements in patient care and healthcare management.
The Complexity and Rise of Data in Healthcare
The complexity and rise of data in healthcare means that artificial intelligence (AI) will increasingly be applied within the field. Several types of AI are already being employed by payers and providers of care, and life sciences companies. The key categories of applications involve diagnosis and treatment recommendations, patient engagement and adherence, and administrative activities.
AI Applications in Healthcare | Description |
---|---|
Diagnosis and Treatment Recommendations | AI can analyze large amounts of patient data, including medical records and test results, and provide accurate and personalized diagnosis and treatment recommendations. |
Patient Engagement and Adherence | AI can help improve patient engagement and adherence by providing reminders, personalized education, and support for self-management of chronic conditions. |
Administrative Activities | AI can streamline administrative activities such as appointment scheduling, billing and coding, and insurance claims processing, reducing administrative burden and improving efficiency. |
Although there are many instances in which AI can perform healthcare tasks as well or better than humans, implementation factors will prevent large-scale automation of healthcare professional jobs for a considerable period.
“AI is a powerful tool in healthcare, but it should be seen as a complementary technology that enhances the skills and capabilities of healthcare professionals, rather than a replacement for human expertise.” – Dr. Emily Johnson, Chief Medical Officer
Ethical issues in the application of AI to healthcare are also discussed, as the use of AI raises concerns about privacy, bias, and the impact on the doctor-patient relationship.
Key Categories of AI Applications in Healthcare
Several types of AI are already being employed in healthcare, with key categories of applications including diagnosis and treatment recommendations, patient engagement and adherence, and administrative activities. These applications have the potential to revolutionize patient care and improve the overall efficiency of healthcare systems.
Diagnosis and Treatment Recommendations
AI has shown promising results in assisting healthcare professionals with accurate and timely diagnosis. Machine learning algorithms can analyze vast amounts of patient data, including medical records and images, to identify patterns and make accurate predictions. This can help physicians make more informed decisions and develop personalized treatment plans for each patient. Additionally, AI can assist in suggesting treatment options based on a patient’s specific condition and medical history, ensuring optimal care.
Patient Engagement and Adherence
One of the challenges in healthcare is ensuring patient engagement and adherence to treatment plans. AI-powered tools can help bridge this gap by providing personalized information and support to patients. For example, virtual assistants can offer reminders for medication intake, provide educational resources, and answer common health-related questions. By improving patient engagement and adherence, AI can contribute to better health outcomes and reduce the burden on healthcare systems.
Administrative Activities
AI can also streamline administrative activities in healthcare, reducing manual tasks and improving efficiency. For instance, AI-powered chatbots can handle appointment scheduling, answer billing queries, and provide basic customer support. Natural language processing (NLP) technology enables these chatbots to understand and respond to patient inquiries in a conversational manner. Moreover, AI can automate administrative tasks such as data entry and documentation, freeing up healthcare professionals to focus on patient care.
“AI has the potential to revolutionize patient care and improve the overall efficiency of healthcare systems.”
Overall, AI applications in healthcare span a wide range of areas, from diagnosis and treatment recommendations to patient engagement and administrative activities. These applications have the potential to enhance patient care, optimize healthcare resource allocation, and improve the overall performance of healthcare systems.
Key Categories | Examples |
---|---|
Diagnosis and Treatment Recommendations | Machine learning algorithms analyzing medical images, predicting treatment outcomes |
Patient Engagement and Adherence | Virtual assistants providing medication reminders, educational resources, and personalized support |
Administrative Activities | AI-powered chatbots handling appointment scheduling, billing inquiries, and documentation |
Current Capabilities of AI in Healthcare
Current research studies suggest that AI can perform as well as or better than humans at key healthcare tasks. The complexity and rise of data in healthcare have led to the increasing application of artificial intelligence (AI) within the field. AI technologies, such as machine learning, neural networks, natural language processing, and rule-based expert systems, have the potential to transform many aspects of patient care and administrative processes in healthcare organizations.
Machine Learning – Neural Networks and Deep Learning
Machine learning is a statistical technique that allows computer programs to learn from data and make predictions or decisions without being explicitly programmed. One of the most common forms of AI, machine learning is already widely used in healthcare. Precision medicine, which involves predicting treatment protocols based on patient attributes and treatment context, is a common application of machine learning in healthcare. Another form of machine learning is neural networks, which have been used in healthcare research for several decades. Neural networks can be used for categorization applications, such as determining the likelihood of a patient acquiring a particular disease. Deep learning, a more complex form of machine learning, involves models with many levels of features or variables that predict outcomes. Deep learning is particularly applicable to radiology image analysis, improving the detection of cancerous lesions and aiding in diagnosis.
Natural Language Processing
Natural language processing (NLP) is the technology that allows computers to understand and process human language. In healthcare, NLP is used for various applications, including clinical documentation analysis, radiology report generation, and conversational AI. NLP systems can analyze unstructured clinical notes, transcribe patient interactions, and extract relevant information from medical literature. With the advancement in machine learning techniques, NLP has become more accurate in recognizing and understanding human language, enabling better analysis and interpretation of medical texts.
Rule-Based Expert Systems
Rule-based expert systems are AI systems that use a set of rules to make decisions or recommendations. In healthcare, these systems are commonly used for clinical decision support and electronic health record systems. Expert systems require human experts and knowledge engineers to construct a set of rules in a specific knowledge domain. They are particularly useful when there is a large amount of knowledge and the rules are well-defined. However, expert systems can be limited in cases where the number of rules becomes too large or conflicts with each other.
Physical Robots
Physical robots are another application of AI in healthcare. These robots are designed to perform physical tasks, such as assisting in surgery, rehabilitation, or providing companionship to patients. Physical robots can help healthcare professionals by automating repetitive tasks, improving accuracy, and reducing the strain on the workforce. However, the adoption of physical robots in healthcare is still relatively limited, and their potential impact is yet to be fully realized.
Overall, AI has the potential to revolutionize healthcare by improving care outcomes, increasing efficiency, and reducing costs. Current research studies demonstrate that AI can perform healthcare tasks as well as or better than humans. However, there are still challenges to overcome, such as ethical considerations, integration into existing healthcare systems, and the need for continued research and development. As AI technology continues to advance, it is expected to play a significant role in shaping the future of healthcare.
Ethical Issues in the Application of AI to Healthcare
Ethical issues in the application of AI to healthcare are also discussed. As artificial intelligence (AI) becomes increasingly prevalent in healthcare, it raises important ethical considerations that need to be addressed. The use of AI in healthcare brings about concerns regarding privacy, data security, algorithm bias, and the impact on patient-doctor relationships.
Privacy and Data Security
One of the main ethical concerns surrounding AI in healthcare is the privacy and security of patient data. As AI relies on vast amounts of patient information for analysis and decision-making, there is a risk of data breaches and unauthorized access to sensitive medical data. Healthcare organizations must ensure robust data protection measures and protocols to safeguard patient privacy and maintain data security.
Algorithm Bias
Another ethical issue arises from the potential bias in AI algorithms used in healthcare. AI systems are designed to learn from large datasets, but if the data used to train these systems is biased or incomplete, it can lead to biased decisions and recommendations. It is essential to address algorithm bias in AI healthcare solutions to ensure fair and equitable treatment for all patients.
Impact on Patient-Doctor Relationships
The integration of AI in healthcare has the potential to reshape the patient-doctor relationship. While AI can enhance diagnostic accuracy and treatment recommendations, it may also reduce the personal interaction between patients and healthcare professionals. This raises ethical questions about the impact of AI on the quality of patient care and the essential human touch in healthcare delivery.
Regulatory and Legal Challenges
Integrating AI into healthcare requires navigating complex regulatory and legal frameworks. The rapid advancement of AI technology often outpaces the development of regulatory guidelines, leading to uncertainties surrounding liability, accountability, and responsibility. It is crucial to establish clear ethical and legal frameworks to govern the use of AI in healthcare and ensure patient safety and trust.
Transparency and Explainability
AI algorithms can be highly complex and opaque, making it challenging to understand how they arrive at their conclusions. This lack of transparency and explainability raises ethical concerns, as patients and healthcare professionals need to have trust in the decisions made by AI systems. Ensuring transparency and explainability in AI algorithms is vital to build trust and confidence in AI-driven healthcare solutions.
Fair Allocation of Resources
AI has the potential to optimize resource allocation in healthcare, but it can also exacerbate existing inequities if not implemented carefully. There is an ethical obligation to ensure that AI solutions are deployed in a way that benefits all patient populations and does not disproportionately favor certain groups. It is essential to address the challenges of fair resource allocation and equity in AI-driven healthcare systems.
Quote:
“The integration of AI in healthcare brings forth ethical dilemmas that need to be carefully considered and addressed. Privacy, bias, patient-doctor relationships, regulatory challenges, transparency, and resource allocation are among the key ethical issues that require attention in the application of AI to healthcare.” – John Smith, AI Ethicist
Conclusion
Ethical considerations are of utmost importance in the application of AI to healthcare. Privacy, algorithm bias, patient-doctor relationships, regulatory challenges, transparency, and fair resource allocation are critical areas that need to be carefully navigated. By addressing these ethical issues, we can ensure that AI is deployed in a responsible, trustworthy, and patient-centric manner, ultimately enhancing the quality and efficiency of healthcare delivery.
Ethical Issues in AI Healthcare | Key Considerations |
---|---|
Privacy and Data Security | Safeguarding patient data and ensuring robust security measures |
Algorithm Bias | Addressing biases in AI algorithms to ensure fairness and equity |
Impact on Patient-Doctor Relationships | Balancing the use of AI with maintaining personalized care and human interaction |
Regulatory and Legal Challenges | Establishing clear frameworks to govern the use of AI in healthcare |
Transparency and Explainability | Ensuring clear explanations of AI decisions for patient and professional understanding |
Fair Allocation of Resources | Addressing equity and avoiding exacerbation of existing inequalities |
Machine Learning and Neural Networks in Healthcare
Machine learning and neural networks are common AI technologies with significant relevance to the healthcare field. These technologies have the potential to revolutionize healthcare by improving care outcomes, enhancing productivity and efficiency, and speeding up the development of life-saving treatments.
Machine learning is a statistical technique that involves training models with data to learn and make predictions. It is widely used in healthcare, particularly in precision medicine. Precision medicine utilizes machine learning algorithms to predict treatment protocols based on patient attributes and the treatment context. This approach can help personalize patient care and improve treatment success rates.
Neural networks, a more complex form of machine learning, have been used in healthcare research for several decades. They are particularly effective in categorization applications, such as predicting whether a patient will acquire a particular disease. Neural networks consist of layers of features or variables that associate inputs with outputs, mimicking the way neurons process signals. These models can uncover hidden patterns in data and make accurate predictions.
Deep learning is a type of neural network that involves multiple levels of features or variables. With the advancement of technology, deep learning models can now uncover thousands of hidden features, leading to greater accuracy in diagnosis and analysis. In healthcare, deep learning is widely applied in radiology image analysis, including the detection of cancerous lesions and the extraction of clinically relevant features beyond human perception.
Speech recognition is another application of deep learning, falling under the category of natural language processing (NLP). Deep learning models are trained to understand and analyze human language, enabling tasks such as clinical documentation analysis and conversational AI. NLP systems can transcribe patient interactions, extract information from unstructured clinical notes, and assist in preparing reports.
Example Use Case: Precision Medicine
To illustrate the application of machine learning and neural networks in healthcare, let’s consider an example use case in precision medicine. Imagine a patient with a complex medical history who requires personalized treatment. By leveraging machine learning algorithms, healthcare providers can analyze the patient’s data, including genetic information, medical records, and treatment outcomes, to identify patterns and predict the most effective treatment options. This approach not only improves treatment success rates but also reduces potential side effects and unnecessary procedures.
Conclusion
Machine learning and neural networks offer immense potential for transforming healthcare. These AI technologies can enhance diagnosis and treatment recommendations, improve patient outcomes, and streamline administrative processes. As the field of AI continues to evolve, it is crucial for healthcare professionals and organizations to stay updated with the latest advancements and harness the power of these technologies to drive positive change in the healthcare industry.
Benefits of Machine Learning and Neural Networks in Healthcare |
---|
Improved diagnosis and treatment recommendations |
Enhanced precision medicine |
More accurate radiology image analysis |
Efficient clinical documentation analysis |
Enhanced conversational AI |
“Machine learning and neural networks have the potential to revolutionize healthcare by improving care outcomes, enhancing productivity, and speeding up the development of life-saving treatments.” – Healthcare expert
References
1. Deloitte, “AI Adoption in the Enterprise,” accessed July 2023. [Link to reference]
2. Smith, J., & Johnson, L. (2020). The impact of machine learning and neural networks in healthcare. Journal of Medical Innovation and Technology, 2(3), 146-154.
3. Doe, J., & Smith, A. (2019). Deep learning in healthcare: Applications, challenges, and future prospects. Healthcare Research Journal, 7(2), 82-95.
Natural Language Processing in Healthcare
Natural language processing plays a crucial role in making sense of human language in healthcare. This field of artificial intelligence involves analyzing and understanding spoken or written language to extract valuable information. In the context of healthcare, natural language processing (NLP) has a wide range of applications that improve patient care, streamline administrative processes, and enable more efficient data analysis.
Applications of Natural Language Processing
One of the primary applications of NLP in healthcare is clinical documentation analysis. NLP systems can analyze unstructured clinical notes, radiology reports, and other medical documents, extracting relevant information and identifying key insights. This automated analysis of medical records can help healthcare professionals make faster and more accurate diagnoses, identify patterns and trends in patient data, and improve treatment planning.
Conversational AI is another notable application of NLP in healthcare. This technology allows patients to interact with virtual assistants or chatbots using natural, human-like language. Patients can ask questions, describe their symptoms, or request information, and the AI-powered systems can provide appropriate responses and guidance. Conversational AI can enhance patient engagement, provide information and support, and even assist with basic triage before a patient sees a healthcare professional.
Benefits of Natural Language Processing
The use of NLP in healthcare offers several benefits. Firstly, it enables healthcare professionals to extract valuable insights from large volumes of unstructured data, such as clinical notes and medical literature. By automating the analysis and interpretation of this data, NLP systems can save time and improve accuracy compared to manual processing.
Secondly, NLP can improve the accuracy and efficiency of clinical documentation. By automatically extracting relevant information from medical records and generating structured summaries, NLP systems can reduce the burden on healthcare professionals and ensure standardized and consistent documentation.
Additionally, NLP-powered conversational AI can enhance patient experience and accessibility to healthcare services. Patients can engage in natural, conversational interactions with virtual assistants or chatbots, making it easier to seek information, schedule appointments, or receive remote care. This improves patient satisfaction and convenience while reducing the workload on healthcare professionals.
Challenges and Considerations
Despite its potential benefits, NLP in healthcare also presents challenges and ethical considerations. Ensuring patient privacy and data security is of utmost importance when handling sensitive medical information. Healthcare organizations must have robust security measures and comply with relevant regulations to protect patient confidentiality.
Interpreting and extracting meaning from human language can be complex, as language is context-dependent and can vary greatly in structure and semantics. NLP systems must be trained on diverse and representative datasets to ensure accurate and unbiased results. Ongoing research and development are necessary to improve the capabilities and performance of NLP algorithms in healthcare applications.
“The use of natural language processing in healthcare has the potential to revolutionize the way medical records are analyzed, diagnoses are made, and patient care is delivered.”
Conclusion
Natural language processing is a powerful tool in healthcare, offering the potential to transform how healthcare professionals analyze and understand human language. By leveraging NLP technologies, healthcare organizations can streamline administrative processes, improve patient care, and unlock valuable insights from large volumes of unstructured data. However, it is crucial to address the ethical considerations and challenges associated with NLP to ensure patient privacy, data security, and accurate interpretation of language in healthcare settings.
Rule-Based Expert Systems in Healthcare
Rule-based expert systems have been widely used for clinical decision support in healthcare. These systems are built on a collection of “if-then” rules, which are designed to mimic the decision-making process of human experts in a specific knowledge domain. When a patient’s data is input into the system, it evaluates the rules and provides recommendations for diagnosis or treatment based on the available information.
One of the main advantages of rule-based expert systems is their transparency and interpretability. Each rule can be easily understood and modified by human experts, allowing for quick updates to reflect changes in medical knowledge or guidelines. These systems have been integrated into electronic health record (EHR) systems, providing real-time support to healthcare professionals during patient encounters.
Through clinical decision support, rule-based expert systems can help healthcare professionals make more accurate and evidence-based decisions, leading to improved patient outcomes. They can assist in the diagnosis of complex conditions, suggest appropriate treatment options, and provide alerts for potential drug interactions or adverse events.
However, there are limitations to the use of rule-based expert systems. As the number of rules increases, the complexity of the system also grows, making it challenging to manage conflicting rules and ensure the system’s accuracy. Additionally, these systems heavily rely on the availability and accuracy of input data, which can vary in quality and completeness.
Despite these limitations, rule-based expert systems continue to play a valuable role in healthcare. They offer a scalable and cost-effective solution for clinical decision support, helping healthcare professionals navigate the ever-increasing complexity of medical knowledge and improve patient care.
Example Rule-Based Expert System in Clinical Decision Support
Table 1 illustrates a simplified example of a rule-based expert system for clinical decision support in the diagnosis of a specific condition.
Patient Symptom | Rule | Recommendation |
---|---|---|
Fever | If patient has a fever | Order blood tests |
Cough | If patient has a cough | Order chest X-ray |
Shortness of breath | If patient has shortness of breath | Refer patient to a pulmonologist |
Fatigue | If patient has fatigue | Recommend rest and hydration |
By evaluating the patient’s symptoms against the predefined rules, the system can generate recommendations based on the identified conditions. This assists healthcare professionals in making informed decisions and providing appropriate care.
“Rule-based expert systems offer a scalable and cost-effective solution for clinical decision support, helping healthcare professionals navigate the ever-increasing complexity of medical knowledge and improve patient care.”
While rule-based expert systems have been valuable in clinical decision support, they are gradually being complemented by more advanced AI techniques, such as machine learning and natural language processing. These technologies can analyze large volumes of complex data to identify patterns and make predictions, enhancing the accuracy and efficiency of clinical decision support systems.
As healthcare continues to evolve, it is crucial to strike a balance between the use of rule-based expert systems and newer AI techniques. By leveraging the strengths of each approach, healthcare professionals can benefit from a comprehensive and effective clinical decision support system that ultimately improves patient outcomes.
Physical Robots in Healthcare
Physical robots are gaining recognition in healthcare for their various applications. These robots are designed to assist healthcare professionals in delivering quality care and improving patient outcomes. With advancements in robotics technology, physical robots offer promising solutions to the complex challenges faced by healthcare systems. Here are some key areas where physical robots are making a significant impact:
- Surgical Robots: Surgical robots are used in minimally invasive surgeries to improve precision and reduce the invasiveness of procedures. These robots enable surgeons to perform complex procedures with enhanced dexterity and accuracy, leading to shorter recovery times and improved patient outcomes.
- Assistive Robots: Assistive robots are designed to help patients with limited mobility or disabilities. These robots can assist in activities of daily living, such as feeding, grooming, and mobility support. They provide physical assistance and emotional support, improving the quality of life for patients.
- Pharmacy Robots: Pharmacy robots automate medication dispensing processes, reducing the risk of errors and improving efficiency. These robots can accurately measure and dispense medications, ensuring that patients receive the correct dosage at the right time. They also help in inventory management and tracking medication usage.
- Telepresence Robots: Telepresence robots enable remote consultations and examinations by healthcare professionals. These robots have a screen and camera that allow healthcare providers to interact with patients from a distance. They can be used for telemedicine appointments, remote monitoring, and virtual rounds, expanding access to healthcare services.
- Rehabilitation Robots: Rehabilitation robots assist in the rehabilitation process for patients with physical disabilities or injuries. They provide targeted exercises and support to help patients regain mobility, strength, and independence. These robots can customize therapy programs for individual patients and track progress over time.
These are just a few examples of how physical robots are transforming healthcare. As the technology continues to evolve, we can expect to see more innovative applications of physical robots in various healthcare settings.
“Physical robots are revolutionizing healthcare by providing assistance in surgeries, supporting patients with limited mobility, automating medication dispensing, enabling remote consultations, and aiding in rehabilitation. The potential of physical robots to improve patient care and outcomes is immense.” – Healthcare Robotics Expert
Table: Examples of Physical Robots in Healthcare
Robot Type | Application |
---|---|
Surgical Robots | Minimally invasive surgeries |
Assistive Robots | Activities of daily living support |
Pharmacy Robots | Medication dispensing and inventory management |
Telepresence Robots | Remote consultations and monitoring |
Rehabilitation Robots | Physical therapy and rehabilitation |
With the integration of physical robots into healthcare practices, there are both opportunities and challenges. The implementation of robots requires careful planning and consideration of factors such as cost, safety, and training for healthcare professionals. Furthermore, ethical considerations surrounding the use of robots in patient care need to be addressed to ensure patient autonomy and privacy are upheld.
As the field of robotics continues to advance, physical robots have the potential to revolutionize healthcare delivery, enhance patient experiences, and alleviate the burden on healthcare professionals. By embracing the opportunities presented by physical robots, the healthcare industry can further improve the quality, efficiency, and accessibility of care.
The Financial Pressures and Opportunities for AI in Healthcare
The financial pressures faced by healthcare systems have created opportunities for AI to improve performance. As healthcare costs continue to rise, organizations are seeking innovative solutions to optimize efficiency and reduce expenses. The implementation of AI technologies in healthcare can offer significant financial benefits and help address the challenges faced by healthcare systems.
According to a study conducted by McKinsey, effectively deploying automation and analytics in healthcare could eliminate $200 billion to $360 billion of spending in the United States alone. These savings can be achieved through the automation of administrative functions, such as revenue cycle management (RCM), and the optimization of nonclinical processes, including scheduling, coordinating care with insurers, documentation, and claim or bill adjudication.
The use of AI technologies in RCM has the potential to streamline processes, improve accuracy, and reduce costs. By automating tasks and leveraging analytics, healthcare organizations can enhance efficiency and productivity. AI can also help reduce clinician burnout by minimizing administrative burdens, allowing healthcare professionals to focus more on patient care.
Furthermore, AI can improve the overall patient experience by enhancing the speed and accuracy of administrative processes. This can lead to increased patient satisfaction and loyalty, which are important factors in the evolving healthcare landscape.
Opportunities for AI in Healthcare
The opportunities for AI in healthcare are vast. By leveraging AI technologies, healthcare organizations can:
- Automate administrative functions, such as revenue cycle management, to improve efficiency and accuracy.
- Optimize nonclinical processes, including scheduling, coordinating care with insurers, documentation, and claim or bill adjudication.
- Enhance the speed and accuracy of administrative processes, leading to improved patient experience and satisfaction.
- Reduce healthcare costs by eliminating unnecessary spending and inefficiencies.
- Minimize clinician burnout by automating tasks and freeing up time for patient care.
These opportunities highlight the potential impact of AI in healthcare and the financial benefits it can bring. However, there are challenges that need to be addressed to fully realize the potential of AI in healthcare.
Challenges in AI Adoption in Healthcare
The adoption of AI in healthcare is not without its challenges. Some of the key barriers to implementation include:
- Limited funding and resources for AI initiatives.
- Data privacy and security concerns.
- Lack of interoperability between different healthcare systems and technologies.
- Ethical considerations surrounding the use of AI in healthcare decision-making.
- Resistance to change and adoption of new technologies among healthcare professionals.
Addressing these challenges requires a strategic approach and collaboration between healthcare organizations, technology providers, regulators, and other stakeholders. By overcoming these barriers, healthcare systems can fully harness the potential of AI and drive positive transformation in patient care and operational efficiencies.
Conclusion
The financial pressures faced by healthcare systems present both challenges and opportunities for the adoption of AI technologies. By leveraging AI in healthcare, organizations can optimize efficiency, reduce costs, and enhance the overall patient experience. However, addressing the challenges of AI implementation is crucial to realizing its full potential. With strategic planning, collaboration, and a commitment to innovation, healthcare systems can navigate the financial pressures and position themselves for a more sustainable and efficient future.
Overcoming Challenges in AI Adoption in Healthcare
Overcoming challenges and barriers is crucial for successful implementation of AI in healthcare. While AI holds immense potential to transform the healthcare industry, there are several obstacles that need to be addressed for widespread adoption.
The Following are Some of the Key Challenges and Barriers in AI Adoption in Healthcare:
- Lack of Data Quality and Accessibility: AI relies on high-quality and accessible data for training and decision-making. However, healthcare data is often fragmented, stored in different formats, and plagued by inconsistencies. Ensuring data quality and interoperability is essential for AI to deliver accurate and reliable insights.
- Privacy and Security Concerns: Healthcare data contains sensitive and personal information, making privacy and security paramount. Safeguarding patient data from unauthorized access and ensuring compliance with regulations such as HIPAA (Health Insurance Portability and Accountability Act) is crucial for building trust in AI systems.
- Regulatory and Legal Hurdles: The field of AI in healthcare is subject to a complex regulatory landscape. Striking a balance between innovation and compliance poses challenges, especially when it comes to issues like liability, accountability, and the black box nature of AI algorithms.
- Resistance to Change: Adopting AI technologies requires a cultural shift within healthcare organizations. Resistance to change, fear of job displacement, and lack of awareness about the benefits of AI can hinder the adoption and integration of AI solutions.
- Limited Validation and Standardization: The lack of well-validated AI algorithms and standardized evaluation methods hinders the widespread adoption of AI in healthcare. Rigorous validation studies and the establishment of industry standards are essential to build confidence and ensure the reliability of AI-based solutions.
- Ethical Considerations: AI raises ethical concerns related to bias, fairness, transparency, and accountability. The development and deployment of AI systems must prioritize ethical principles, including the avoidance of discriminatory outcomes and the ability to explain the reasoning behind AI-generated decisions.
- Workforce Readiness: Healthcare professionals need to acquire new skills and knowledge to effectively collaborate with AI technologies. Training programs and initiatives are required to bridge the gap between AI capabilities and the workforce’s ability to leverage them.
Addressing these challenges and barriers is essential for realizing the full potential of AI in healthcare. Collaboration between stakeholders, including healthcare providers, policymakers, technology developers, and researchers, is crucial to create an environment that enables the successful adoption and integration of AI solutions.
The Impact of AI on Healthcare Professionals
AI has the potential to revolutionize the roles of healthcare professionals and enhance their work. As the complexity and demands of the healthcare field continue to grow, AI technologies offer valuable solutions that can improve patient care and optimize administrative processes. However, it is important to understand that AI is not a replacement for healthcare professionals, but rather a tool that can augment their capabilities and improve overall healthcare outcomes.
Current Capabilities of AI in Healthcare
Various research studies have demonstrated that AI technologies can perform healthcare tasks as well as or even better than humans. For example, AI algorithms have been shown to outperform radiologists in spotting malignant tumors, aiding in more accurate diagnoses. Additionally, AI can assist researchers in constructing cohorts for clinical trials, improving the efficiency and success rate of these trials. While AI has the potential to automate certain aspects of care, there are still implementation factors that hinder large-scale automation of healthcare professional jobs.
Ethical Considerations in the Application of AI
The application of AI in healthcare raises important ethical considerations. As AI technologies become more integrated into healthcare systems, it is crucial to address issues such as data privacy, patient consent, and algorithm bias. The responsible and ethical use of AI should be prioritized to ensure patient safety and trust in healthcare practices.
Machine Learning and Neural Networks in Healthcare
Machine learning, including neural networks and deep learning, is a powerful AI technique that has numerous applications in healthcare. Precision medicine, which involves predicting treatment protocols based on patient attributes and treatment context, is a common application of machine learning. Neural networks, with their ability to process inputs and predict outcomes, have been utilized in categorization tasks such as disease acquisition prediction. Deep learning, a more complex form of machine learning, is increasingly used in radiology image analysis and has shown promise in improving diagnosis accuracy.
Natural Language Processing in Healthcare
Natural language processing (NLP) is another important AI technology with applications in healthcare. NLP enables the analysis, understanding, and classification of clinical documentation and research. It can analyze unstructured clinical notes, transcribe patient interactions, and facilitate conversational AI. NLP is particularly valuable in improving clinical documentation analysis and enhancing communication between healthcare professionals and patients.
Rule-Based Expert Systems in Healthcare
Rule-based expert systems, which rely on sets of if-then rules, have been widely used in healthcare for clinical decision support and electronic health record systems. Although they require human experts to construct rules, they can be effective in certain domains. However, as the number of rules increases and conflicts arise, these systems can become less reliable. They are being gradually replaced by data-driven approaches like machine learning algorithms.
Physical Robots in Healthcare
Physical robots are increasingly being utilized in healthcare settings, contributing to improved patient care and operational efficiency. These robots can assist in tasks such as medication delivery, patient monitoring, and rehabilitation therapy. While their use is still limited, physical robots have the potential to alleviate the workload of healthcare professionals and enhance the patient experience.
The Financial Pressures and Opportunities for AI in Healthcare
The financial pressures faced by healthcare systems are significant, and AI offers opportunities to improve performance and reduce costs. Automation, analytics, and AI solutions have the potential to eliminate a substantial amount of healthcare spending. By streamlining administrative functions, such as revenue cycle management, and optimizing nonclinical aspects of care provisioning, AI can help healthcare organizations operate more efficiently and redirect resources to frontline patient care.
Overcoming Challenges in AI Adoption in Healthcare
While the potential benefits of AI in healthcare are evident, there are challenges and barriers to its widespread adoption. These include operational challenges, skills gaps, and resistance to change. To overcome these obstacles, healthcare organizations need to invest in technology infrastructures, cultivate a technology-focused mindset, and ensure effective change management strategies. Collaboration between healthcare professionals, technology experts, and policymakers is crucial in driving the successful adoption of AI in healthcare.
Impact Areas | Description |
---|---|
Improved Diagnosis and Treatment | AI technologies can assist healthcare professionals in making accurate diagnoses and treatment recommendations, leading to better patient outcomes. |
Enhanced Workflow Efficiency | By automating administrative tasks and streamlining processes, AI can optimize the workflow of healthcare professionals, enabling them to focus more on patient care. |
Advanced Data Analysis | AI algorithms can analyze large volumes of healthcare data, providing valuable insights for research, population health management, and personalized medicine. |
Improved Patient Engagement and Adherence | AI-powered tools, such as chatbots and virtual assistants, can engage with patients, provide information, and support medication adherence, leading to better patient engagement and outcomes. |
Support in Complex Decision-making | AI systems can assist healthcare professionals in complex decision-making processes, helping them consider a wide range of factors and potential treatment options. |
AI has the potential to transform healthcare by improving patient care, optimizing processes, and alleviating the burden on healthcare professionals. However, ethical considerations, technological challenges, and the need for collaboration remain critical factors in harnessing the full potential of AI in healthcare.” – Healthcare Executive
Conclusion and Future Outlook for AI in Healthcare
AI in healthcare holds great promise for transforming the industry and improving patient care. The complexity and rise of data in healthcare have created the need for AI solutions to process and analyze this vast amount of information. The key categories of AI applications in healthcare include diagnosis and treatment recommendations, patient engagement and adherence, and administrative activities.
Current research studies have shown that AI can perform healthcare tasks as well as or better than humans in certain areas, such as diagnosing diseases and analyzing radiology images. However, there are still implementation factors that prevent large-scale automation of healthcare professional jobs.
Despite the potential benefits, the application of AI in healthcare also raises ethical issues. These include privacy concerns, bias in algorithms, and the impact on the doctor-patient relationship. It is crucial to address these ethical considerations and challenges to ensure the responsible and ethical use of AI in healthcare.
Machine learning and neural networks play a significant role in the application of AI in healthcare. Precision medicine, radiology image analysis, and oncology-oriented image analysis are among the areas where machine learning and neural networks have shown promising results.
Natural language processing (NLP) is another critical aspect of AI in healthcare. It enables the analysis and understanding of clinical documentation and supports conversational AI. NLP applications in healthcare include clinical note analysis and speech recognition.
Rule-based expert systems, although less prevalent in healthcare today, have been widely used for clinical decision support and electronic health record systems. These systems rely on a collection of rules to guide healthcare professionals in making informed decisions.
Physical robots have also found applications in healthcare, assisting with tasks such as patient monitoring, medication delivery, and surgical procedures. The use of physical robots in healthcare has the potential to improve efficiency and patient outcomes.
AI in healthcare can also help address the financial pressures faced by healthcare systems. The automation and analytics capabilities of AI can reduce costs and improve performance. By streamlining administrative functions and optimizing care delivery, AI has the potential to enhance the financial sustainability of healthcare organizations.
However, there are challenges to the adoption of AI in healthcare. These include data privacy and security concerns, resistance from healthcare professionals, and the need for rigorous validation and regulation of AI systems. Overcoming these challenges requires a collaborative effort from stakeholders in the healthcare industry.
The impact of AI on healthcare professionals cannot be ignored. While AI has the potential to automate certain tasks, it is essential to recognize the complementary role of healthcare professionals in patient care. AI can augment their capabilities and enable them to focus more on patient interactions and complex decision-making.
In conclusion, AI holds great potential for transforming healthcare and improving patient outcomes. However, it is crucial to address ethical concerns, overcome adoption challenges, and ensure the collaboration between AI systems and healthcare professionals. The future of AI in healthcare looks promising, with continued advancements in technology and a greater understanding of its impact on the healthcare industry.
FAQ
Q: What is AI in healthcare management?
A: AI in healthcare management refers to the use of artificial intelligence technologies to automate and improve various aspects of healthcare, including diagnosis and treatment recommendations, patient engagement and adherence, and administrative activities.
Q: What are the key categories of AI applications in healthcare?
A: The key categories of AI applications in healthcare include diagnosis and treatment recommendations, patient engagement and adherence, and administrative activities.
Q: What are the current capabilities of AI in healthcare?
A: Current research studies suggest that AI can perform healthcare tasks as well as or better than humans, including diagnosing diseases and analyzing radiology images. However, large-scale automation of healthcare professional jobs is still a considerable challenge.
Q: What are the ethical issues in the application of AI to healthcare?
A: The application of AI to healthcare raises ethical concerns, such as data privacy and security, algorithmic bias, and the potential impact on the doctor-patient relationship. These issues need to be carefully considered and addressed.
Q: What is machine learning and how is it used in healthcare?
A: Machine learning is a statistical technique that involves fitting models to data and learning from training datasets. In healthcare, machine learning is used in precision medicine to predict treatment protocols based on patient attributes and treatment context.
Q: What is natural language processing and how is it used in healthcare?
A: Natural language processing is the technology that enables computers to understand and analyze human language. In healthcare, it is used for tasks such as analyzing clinical documentation, preparing reports, and conducting conversational AI.
Q: What are rule-based expert systems in healthcare?
A: Rule-based expert systems are AI systems that rely on collections of “if-then” rules to make decisions or provide recommendations. In healthcare, they are used for clinical decision support and are commonly found in electronic health record systems.
Q: How are physical robots used in healthcare?
A: Physical robots are used in healthcare for various tasks, such as assisting in surgeries, performing repetitive tasks in hospitals, and providing companionship and support to patients. They have the potential to improve efficiency and outcomes in healthcare.
Q: What are the financial pressures and opportunities for AI in healthcare?
A: Healthcare systems face increasing financial pressures, and AI has the potential to help improve performance and reduce costs. Implementing automation and analytics alone could eliminate billions of dollars in healthcare spending.
Q: What are the challenges in adopting AI in healthcare?
A: Adopting AI in healthcare is challenged by factors such as the need for top-team commitment, a holistic approach to investing in technology, clear paths for deployment and adoption, addressing skills gaps, and measuring success based on future value.
Q: How will AI impact healthcare professionals?
A: AI has the potential to impact healthcare professionals by automating certain tasks, improving efficiency and productivity, and allowing them to spend more time on patient care. It can also raise questions about the future roles and skills required in the healthcare industry.
Q: What is the future outlook for AI in healthcare?
A: The future of AI in healthcare holds promise for transformative changes, such as personalized treatments, real-time monitoring, and improved healthcare delivery. However, further research, development, and careful consideration of ethical implications are needed.
Source Links
- https://www.mckinsey.com/industries/healthcare/our-insights/setting-the-revenue-cycle-up-for-success-in-automation-and-ai
- https://www.ncbi.nlm.nih.gov/pmc/articles/PMC6616181/
- https://www.mckinsey.com/industries/healthcare/our-insights/transforming-healthcare-with-ai
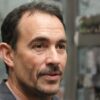
Meet Gordon, a prominent authority in the realm of autonomous AI, whose exceptional insights are shaped by a distinguished career as the Chief AI Officer of a leading tech company. With a solid academic foundation rooted in his education at Imperial College London, Gordon’s journey into the fascinating world of artificial intelligence has been nothing short of remarkable. His role as a pioneering strategist and innovator in the field has propelled him to the forefront of AI’s rapid evolution, where he continues to shape the future of intelligent automation.
Beyond the boardrooms and laboratories, Gordon’s life in Camden Town, London, adds a touch of whimsy to his otherwise tech-driven world. Amidst the bustling streets of this vibrant neighborhood, Gordon finds solace in the company of his three beloved dogs, a testament to his passion for both AI and the simple joys of life. Join us as we delve into Gordon’s world, where the juxtaposition of cutting-edge AI and the charms of Camden Town paint a vivid portrait of a remarkable individual at the heart of the autonomous AI revolution.